Friends,
On Sunday’s Risk Depends On The Resolution, I wrote:
The history of the US stock market is a small sample size. The true sample size requires looking at non-overlapping returns as opposed to rolling 12-month returns. Which means you get as many data points as you do years.
This echoes Euan Sinclair’s point in Positional Option Trading:
In the long term, values are related to macro variables such as inflation, monetary policy, commodity prices, interest rates, and earnings. These change on the order of months and years. Worse still, they are all codependent. A better way to think of market data might be that we are seeing a small number of data points that occur a lot of times. This makes quantitative analysis of historical data much less useful than is commonly thought.
In other words you should be “thinking in N not T” and admonition that acknowledges that samples drawn from the same regime reduce N.
[Plagiarism Disclaimer: I can’t find the origin of that “N not T” phrase but it’s not mine originally.]
This is also why the concept of attractor landscapes is important. Samples drawn from the same basin of activity are correlated to an underlying gravity that perpetuates the basin.
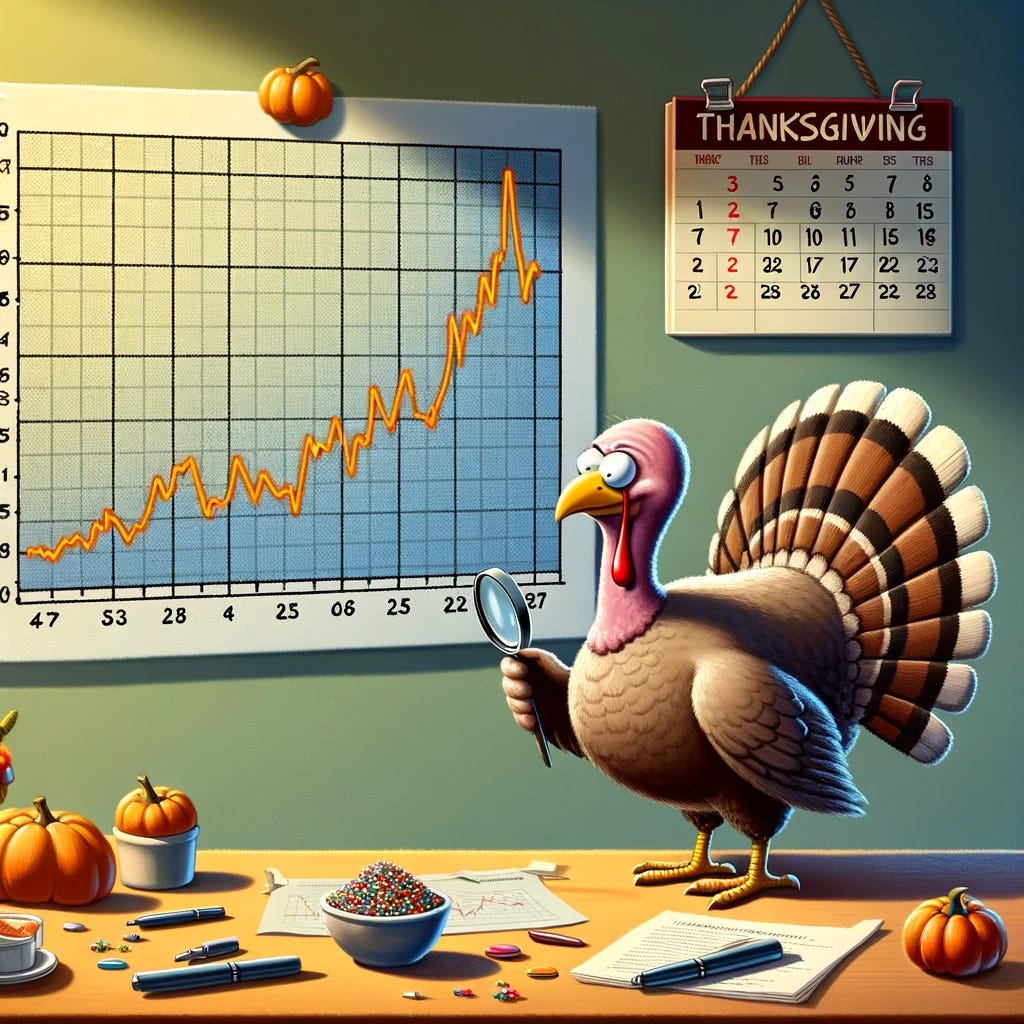
A paper that made the rounds in October, Stocks for the Long Run? Sometimes Yes, Sometimes No describes the “regime thesis” and how it shrinks sample size:
In conventional financial history, greatest weight would be placed on values computed over the longest interval available, on the theory that sampling returns over time follows the same logic as sampling people from groups. Longer time samples ought to provide more precise estimates of the true expected return on an asset, just as a larger sample of people would produce a better estimate of any differences in height or weight across groups.
The regime thesis denies that longer time intervals are like larger samples. It does not assume a population of asset returns existing outside of time from which larger samples can be drawn by lengthening the series. There are only the asset returns that have been recorded in history thus far. These do not predict future asset returns because their pattern is specific to the regime that prevailed at the time. No analysis of US bond returns from 1792 to 1941 could have predicted the bond returns seen following the war—such a bond abyss had never occurred before.
A regime is a temporary pattern of asset returns that may persist for decades. The regime thesis entails no periodicity and requires no reversion…Stationarity prevails within regimes but not across them. The idea of temporary stationarity distinguishes the regime thesis from Pástor and Stambaugh’s idea that the parameters of the return distribution are unknown. Under their thesis, the new 19th-century US data and the country-level international results can be characterized as an expanded sample relative to Ibbotson. The much larger sample better captures the true volatility of stock and bond returns by allowing more opportunities for extremes to emerge—in particular, extremes over multi-decade intervals, which are necessarily few in a single-market, single-century sample. The regime thesis differs in expecting sustained but temporary stationarity. Stocks and bonds ran neck and neck for over a century (Figure 1). Then after the war, that regime gave way to one where stocks beat bonds, year after year, decade after decade.
An adjacent idea, perhaps an instance of the regime construct, is a trend.
I’ll start with an example that vol traders will grumble about because it’s to familiar.
Imagine the trader buys an option implying 20% annual volatility. They delta hedge a long 2-week option once a day at the close but the stock goes up 1% per day.
The daily volatility annualized is 1% x √251 = 15.84%
The weekly volatility annualized is 5% x √52 = 36.1%
They would be better off if they hedged (ie sampled the vol) weekly. The auto-correlation of the moves affects the scaling of volatility from 1 day to 1 week.
The assumption that volatility scales with √n, where n is a unit of time, only holds when the returns are uncorrelated.
Adjusting volatility for auto-correlation
On the CME’s website is an old white paper by Newedge called It’s the autocorrelation, stupid.
Premise
Failing to account for autocorrelated returns can lead to serious biases in our estimates of return volatilities…[in the presence of autocorrelation] the standard square root of time rule that the industry uses to translate daily, weekly, or monthly volatilities into annualized volatilities is wrong and produced biased results.
I gave GPT a break and let Claude summarize the paper:
The paper is looking at how autocorrelation, which is the correlation between a time series and its own past or future values, affects risk measures like volatility and drawdowns for investments.
Two example investments are compared - a global stock index and a commodity trading advisor (CTA) index. Even though they have the same average return and volatility, the stock index has larger and longer drawdowns.
The reason is that the stock returns tend to be positively autocorrelated - if returns are up one month, they tend to also be up the next month. So gains build on gains, and losses build on losses.
The CTA returns tend to be negatively autocorrelated - if returns are up one month, they tend to be down the next. This means drawdowns are shorter for CTAs.
Standard methods for calculating risk don't account for autocorrelation. So they underestimate risk for positively autocorrelated assets like stocks, and overestimate risk for negatively autocorrelated assets like CTAs.
The paper shows how to adjust volatility and drawdown estimates for autocorrelation. This gives better comparisons of risk across different investments.
It also means that common risk-adjusted return measures like the Sharpe ratio can be misleading when autocorrelation isn't considered. The risk-adjusted returns look very different for stocks vs. CTAs after adjusting for autocorrelation.
In summary, the paper shows that accounting for autocorrelation gives better measures of risk and returns for investments.
How to adjust for auto-correlation when translating single-period to multi-period volatilities:
I asked Claude for an intuitive explanation of the 1 + 2 x (autocorrelation) adjustment:
But with autocorrelated returns, variances don't just add up independently. The adjustment accounts for this by multiplying the standard formula by (1 + 2 x Autocorrelation).
If the autocorrelation is positive, the (1 + 2AC) term will be bigger than 1. So the yearly variance will increase compared to the standard calculation.
If autocorrelation is negative, (1 + 2AC) will be less than 1. So the yearly variance will decrease.
Intuitively:
Positive autocorrelation means returns build on themselves - gains lead to more gains, losses to more losses. This increases risk.
Negative autocorrelation means returns tend to reverse - gains are followed by losses. This decreases risk.
The adjustment accounts for this by increasing/decreasing the yearly variance compared to the standard formula.
As an option trader, a grinding (auto-correlated) trend has intuitively felt like looking at realized volatility sampled daily understated the true volatility. To tease it out I could look at realized volatility at different times scales (intra-day, daily, weekly) and consider how the implied volatility was weighing them. The market isn’t stupid…in the earlier example, if the stock has moved 5% in a week with daily 1% moves it’s unlikely that it is going to offer you vol at 16%.
(There’s a nerd reader right now rushing to regress the current IV on various historical realized windows to impute the weights. If there is known event in the rearview, when you mentally discount its effect on your assessment of future realized volatility you are doing this intuitively!)
Now if we could only predict autocorrelation in advance.
I’ll leave you with one last quote from the paper that can be extrapolated as a wider word of caution:
Correlations are volatile so it takes a long time to detect them with confidence.
The choice of a 60-month rolling estimation period was influenced in part by the time it takes to detect autocorrelation with any kind of statistical reliability. The standard deviation of a single correlation estimate when the true correlation is zero is roughly one over the square root of the number of observations, or 1 / √n. With 60 months, the standard deviation would be about 0.13 [= 1 / √60], which means that five years or 60 months is about the amount of time needed to detect an overall correlation of 0.25.
Extra
Claude’s derivation of the adjustment:
Let's say returns follow an autocorrelated process.
Rt = φRt-1 + εt
Where φ
is the autocorrelation coefficient and εt
is white noise.
The variance of Rt
is then:
Var(Rt) = φ²Var(Rt-1) + Var(εt)
Since Var(εt
) is just the 1-period variance σ²
, this becomes:
Var(Rt) = φ²Var(Rt-1) + σ²
Assume variance is constant over time due to stationarity so:
Var(Rt) = Var(Rt-1)
Plugging in:
Var(Rt) = φ²Var(Rt) + σ²
Factoring:
Var(Rt) - φ²Var(Rt) = σ²
(1- φ²) Var(Rt)= σ²
Var(Rt) = σ²/(1 - φ²)
Now suppose we are converting a 1-period (e.g. daily) variance σ² to an annual variance over n periods (e.g. n = 250 trading days).
The annualized multi-period variance is then:
Var(Rannual) = nVar(Rt)
Plugging in the expression for Var(Rt) and simplifying:
Var(Rannual) = n(σ²/(1 - φ²)) =
nσ²(1 + 2φ + φ²)/(1 - φ⁴) ≈
nσ²(1 + 2φ)
Where the last step holds for small φ, with φ² and higher order terms going to 0.
You might notice a little Easter egg on archived technical posts at Moontower. Stay tuned :-)
☮️
Stay groovy!
Need help analyzing a business, investment or career decision?
Book a call with me.
It's $500 for 60 minutes. Let's work through your problem together. If you're not satisfied, you get a refund.
Let me know what you want to discuss and I’ll give you a straight answer on whether I can be helpful before we chat.
I started doing these in early 2022 by accident via inbound inquiries from readers. So I hung out a shingle through the Substack Meetings beta. You can see how I’ve helped others:
Moontower On The Web
📡All Moontower Meta Blog Posts
🌕Welcome Traveler
Specific Moontower Projects
🧀MoontowerMoney
👽MoontowerQuant
🌟Affirmations and North Stars
🧠Moontower Brain-Plug In
Curations
✒️Moontower’s Favorite Posts By Others
🔖Guides To Reading I Enjoyed
🛋️Investment Blogs I Read
📚Book Ideas for Kids
Fun
🎙️Moontower Music
🍸Moontower Cocktails
🎲Moontower Boardgaming